Revolution in manufacturing: Machine learning improves the precision of sheet metal stamping
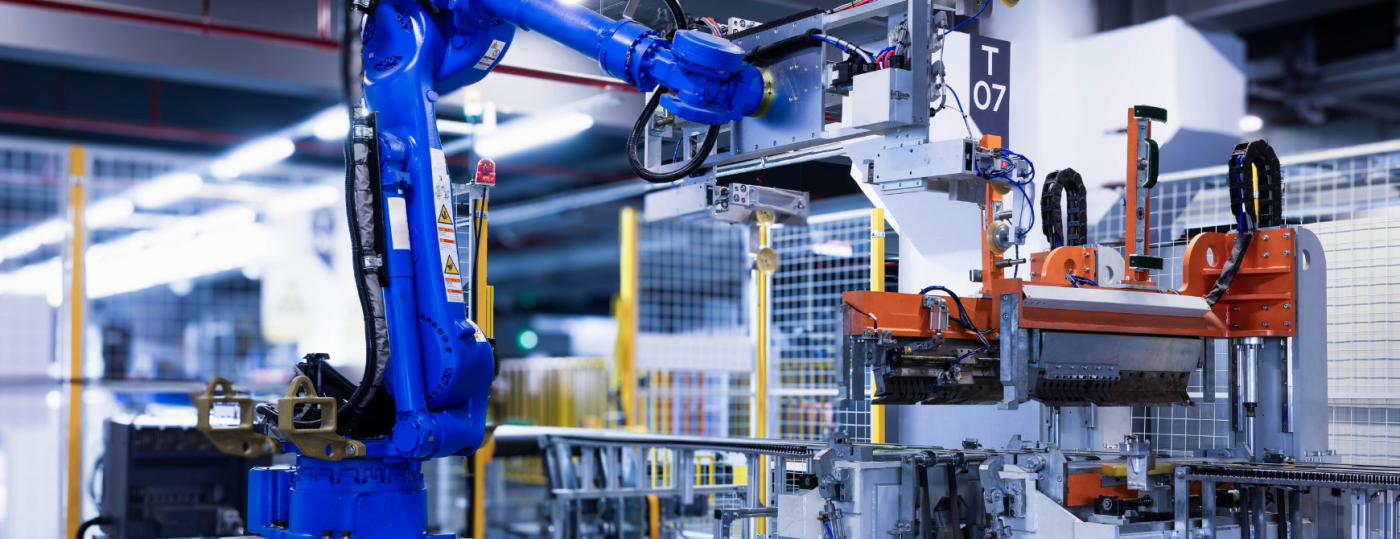
UL FS
Date of publication:
Advancements in sheet metal stamping processes are creating new opportunities in industrial production. Researchers from the Faculty of Mechanical Engineering at the University of Ljubljana have developed an advanced method that uses machine learning to improve accuracy and reduce material waste.
The study presents an advanced machine learning model that combines light gradient boosting machine (LightGBM) and deep neural networks (DNNs) to optimise prediction accuracy in sheet metal stamping. DNNs are computer models that mimic the functioning of the human brain for pattern recognition and decision-making, while LightGBM is an advanced machine learning algorithm optimised to process large amounts of data quickly and accurately. This innovative approach improves manufacturing precision, minimises errors and creates a robust basis for digital twin applications that promote smarter and more efficient production processes. This contributes to reducing waste material, optimising costs and ensuring high product quality, which is crucial for the automotive and aerospace industries, where precision, strict tolerances and reliability are key requirements.
Innovative approaches to more precise stamping
Sheet metal stamping enables the mass production of high-precision sheet metal parts, but maintaining geometric accuracy remains a challenge. It is influenced by material properties, springback effects and process variability. Conventional predictive models are mainly based on finite element method (FEM) simulations, which provide detailed insights into material deformation and process dynamics. However, FEM-based approaches are computationally intensive and require significant processing time to achieve high accuracy, making their real-time implementation impractical in dynamic production environments.
To overcome these limitations, researchers have developed an advanced ensemble model that combines LightGBM and DNNs. By training on extensive FEM-generated datasets, the model captures complex nonlinear interactions between material properties, process parameters and final part geometries. This approach significantly improves predictive accuracy and achieves a high coefficient of determination R² of 0.951, ensuring greater consistency of the stamped part geometry. The model enables decision-making in real time and serves as a fundamental step towards fully digitalised and adaptive manufacturing processes.
"By integrating real-time predictive analytics with advanced machine learning models, our research presents a novel solution that advances digital twin technology and significantly improves decision-making in modern stamping processes," said Assoc. Prof. Dr. Tomaž Pepelnjak and Ema Stefanovska, the authors of the publication.
Real-time monitoring and control
Through the application of this advanced predictive model into real-time monitoring systems, manufacturers can optimise production and improve product quality. This research makes an important contribution to smart manufacturing by enabling the development of digital twins, improving automation and increasing efficiency in the context of Industry 4.0.